The use of multi-cloud environments, B2B automation, mobile apps and APIs have increased. As a result, so has the risk to organisational data. This risk to organisational data makes it imperative to find policy generation solutions to protect your network
Botnets are becoming increasingly intelligent and attack with a variety of vectors. These attacks place a larger strain on your security profile and IT team. Your IT team must keep up with rapidly evolving threats by creating and maintaining security policies to adapt to the bots.
Policy automation can be a solution to these evolving bots. Through machine learning, auto policy generation is a sophisticated security response to these ever-smarter, multi-dimensional cyber attacks. See how Radware’s 360-degree approach to app security reduces risk and speeds up scalable, quality application security.
Common pitfalls of application security
- Using Web Application Firewall (WAF) as a standalone security feature: Non-app based threats must be part of a holistic security strategy. Security threats occur in a variety of forms and all forms must be accounted for.
- Negative security WAF models can’t identify zero-day attacks: This limits protection capacity and quality. This security system relies on the team to remain up to date on exploits as they come out. Since new exploits are being discovered everyday, your data will fall victim as no rule is in place to protect you.
- One security solution across platforms: A lack of tailored system requirements creates system vulnerabilities. Each platform should have a security solution tailored to each of its weak points. This creates a comprehensive solution that addresses how to protect data.
Automating your security policy mapping and generation
No single security feature covers all possible attack vectors. Thus, auto policy generation is a key component of a 360-degree defence.
Manually updating and generating policies to mitigate new security risks is intensive. It is also time-consuming, utilises IT resources and runs the risk of human error. However, automatic policy generation is responsive. It is able to continually implement and update security policies to match evolving threats.
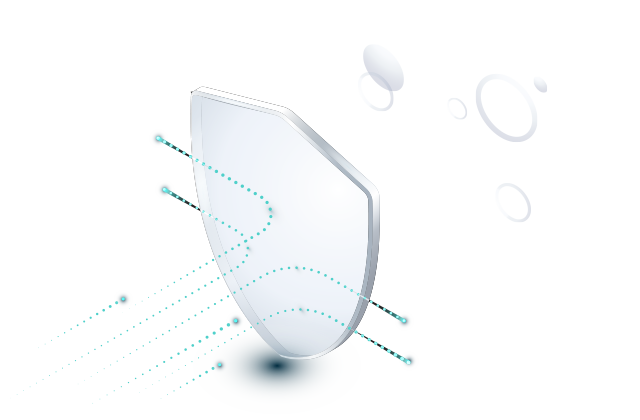
There are 4 steps to auto policy mapping:
- Application mapping
- Threat analysis
- Policy generation
- Policy activation
Auto policy generation leverages machine learning to secure web applications. It requires as little manual input as possible through the use of security filters, traffic analysis, and dynamic network profiles. Radware’s auto policy generation technology enables all rules but creates exceptions to limit false positives.
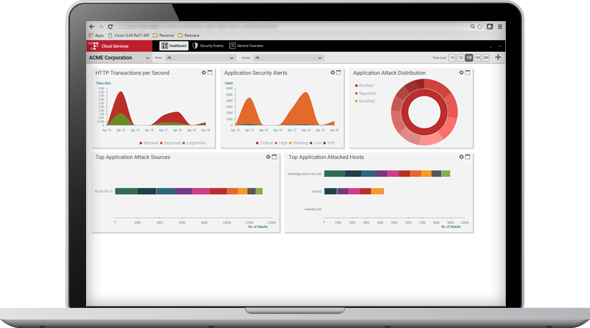
Are you covered for zero-day attacks?
Many security products rely on static negative models. These models only define and maintain disallowed traffic against OWASP Top 10 risks. This means the models can’t recognise zero-day attacks (previously known as unseen attacks) across risk categories. However, a positive security model defines allowable traffic. This limits the traffic allowed in by having set criteria they must fit.
Using both positive and negative models provide benefits from both worlds. As a result, Radware’s auto policy generation encompasses both models to adapt to the threat environment. Subsequently, this secures your system by preventing SQL injection and cross-site scripting (XSS) attacks.
Machine learning builds profiles based on typical use and uses them to support auto-generation. For instance, when an activity doesn’t align, it triggers an alert and security defence mechanisms.
Radware’s AppWall and Cloud WAF Service use auto policy generation. As a result, it automatically uses the required security filter. In addition, it creates security filter rules and switches the security filters into active mode to provide a fast, efficient, and automated security offering.
The evolution of machine learning
The machines aren’t quite independent yet. For example, security experts review automated policies. By doing so, these reviews determine the validity, integrity, and risks of false positives and negatives from the auto generated policies.
There are two key trade-offs in machine learning. In other words, the trade-offs include the complexity of the algorithm and automatic or the time to mitigate. On one hand, the simpler algorithms act faster but are more open to attacks. On the other hand, complex algorithms provide more defence but can take longer to mitigate risks.
Global spending on AI is expected to hit $98 billion by 2023. Therefore, rapid developments in machine learning are expected. In short, it will continue to make automated policy generation a critical part of security profiles. If you’re interested in how else Outcomex uses artificial intelligence, check out our Compliance as a Service solution.
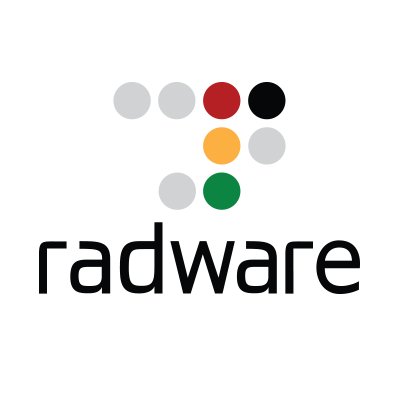
Take a 360 degree view of app security with Radware and Outcomex
Find out how you can harness automated policy generation as part of your security stack. Talk Radware with your account manager or reach out to our sales team at sales@outcomex.com.au.
Download Radware’s Auto Policy Generation Data Sheet
Complete this form to download Radware’s Auto Policy Generation Data Sheet.
In this 4-page document, learn more about the 4 steps of auto policy generation, the human factor behind automation, and how auto policy impacts the quality of protection.